iPC EU Horizon 2020 - Individualized Paediatric Cure: Cloud-based Virtual-patient Models for Precision Paediatric Oncology
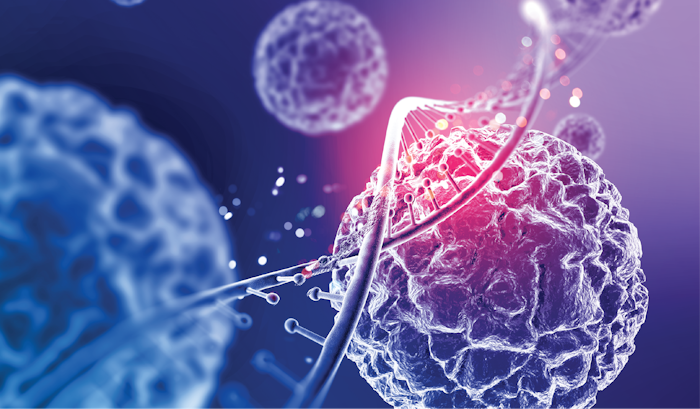
About this
Project
The objective of this proposed research is to advance the field of unsupervised visual anomaly detection (UAD), with a focus on medical imagery. Unsupervised visual anomaly detection is a data analysis method used to model and detect previously unknown anomalies in data, streamlining the process of tumor research and treatment development. Developed UAD methods will be evaluated on all the available medical imagery available as part of the Pediatric Brain Tumor Atlas such as histology images, radiology images, and MR images. In comparison with existing supervised approaches, unsupervised approaches will be widely applicable to the vast amount of existing medical imagery data. Research will be performed as part of the iPC EU Horizon 2020 project1, where one of the main objectives of the proposed research is to support individualized diagnostics and treatment for pediatric brain cancer patients.
Ask The
Scientists
What are the goals of this project?
The goals of this project include advancing cancer diagnostics through the application of unsupervised visual anomaly detection on medical imagery.
What is the impact of this project?
UAD methods will support the individualized treatment of pediatric brain cancers by streamlining the process of diagnosis. These methods will also be applicable to the analysis of all medical imagery.
Why is the CBTN request important to this project?
UAD methods will support the individualized treatment of pediatric brain cancers by streamlining the process of diagnosis. These methods will also be applicable to the analysis of all medical imagery.
Specimen Data
The Children's Brain Tumor Network contributed to this project by providing access to the Pediatric Brain Tumor Atlas.
Meet The
Team
PI: Dejan Stepec
Danijel Skocaj
related